Xiao Li
Assistant Professor
School of Data Science
The Chinese University of Hong Kong, Shenzhen
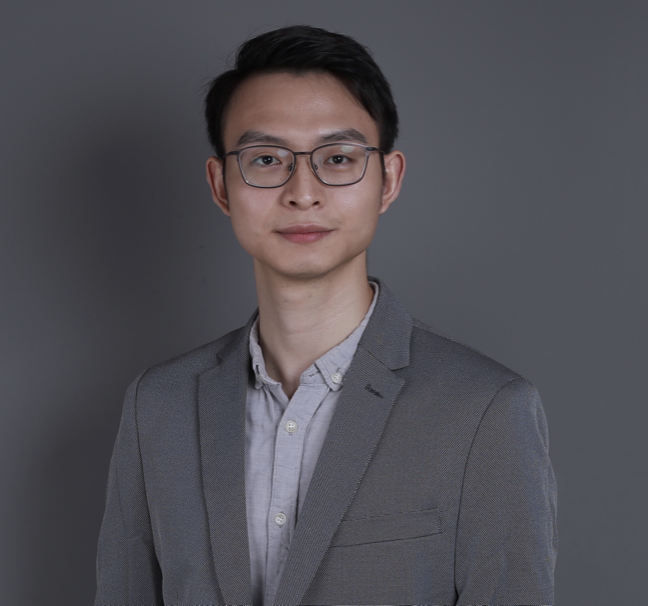
A little about me: Starting from summer 2020, I am an Assistant Professor at the School of Data Science at The Chinese University of Hong Kong, Shenzhen. Before that, I obtained my Ph.D. degree at The Chinese University of Hong Kong from 2016 to 2020. My superviors are Prof. Thierry Blu and Prof. Anthony Man-Cho So. I did my undergraduate at Zhejiang University of Technology from 2012 to 2016.
Contact: lixiao at cuhk.edu.cn
Office: 506a, Daoyuan Building, The Chinese University of Hong Kong, Shenzhen.
Abstract (of research): I work on the intersection of (continuous) optimization and machine leanring. In particular, I am interested in designing and analyzing deterministic and stochastic optimization algorithms, and analyzing specific nonconvex (and possibly nonsmooth) formulations arising in machine learning and signal processing. I wish to reveal simple ideas and results. See the “research” page for more detials.
I would give my deep thanks to my collaborators and students. I highly appreciate the funding agencies that supported my research such as NSFC, RGC, Shenzhen Science and Technology Program, etc.
Prospective Students
I am seeking one PhD student who is self-motivated and has a solid background in mathematics and programming. If you’re interested, please email me your CV and any other supporting documents.
The original theme template is al-folio.